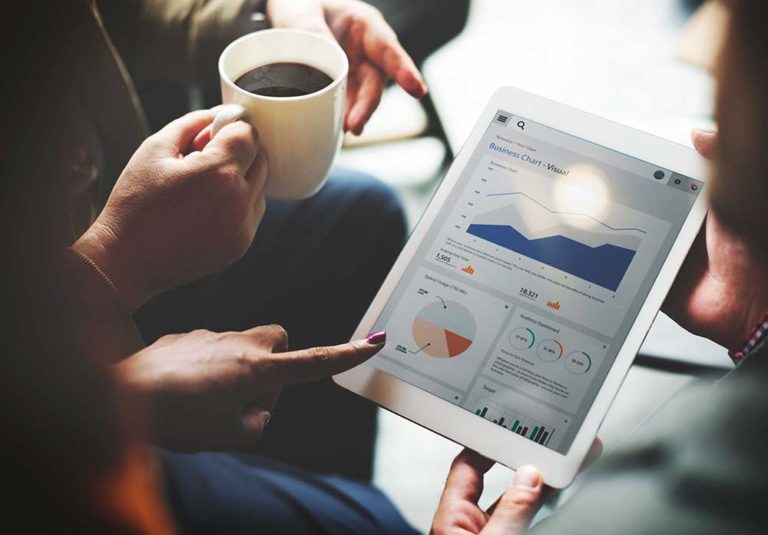
External factors constantly affect your company and the sales, operational, marketing, staffing, and planning decisions you make on a day-to-day basis. No company operates in a vacuum; all are subject to environmental changes that are outside of their control. And while I wish this was an article about how to adjust those external factors to fit your business plan, that is rarely possible. Instead, what you can do is incorporate these factors into your decision-making process by incorporating them into your analytics and business intelligence.
Most companies have turned to data analytics, statistical modeling, prescriptive analytics, and artificial intelligence to inform business decisions; but many models are built only from internal data, analyzing how these internal components are trending over time or seasons. When models don’t account for the complexities of external events, there is potential for false positives or negatives and result in a lack of comprehensive understanding of the causes of these outcomes. Therefore, control data is an incredibly important, but often overlooked, component of applied data science.
What is Control Data?
Control data is data about external factors that impact the outcome of variables in a model. Control data is often referred to as external data or third-party data. Using control data in a model allows data scientists to figure out which factors outside of the business have affected outcomes in the past, map out the correlation between those factors, and inform how the business may perform in the future due to these same factors. Of course, correlation does not always prove causation, so it is important for humans (rather than solely AI) to analyze information for a deep understanding of how and why these factors have a correlation in the first place.
Examples of control data include economic trends, weather, demographic trends, COVID-19 numbers, government regulations, population growth or decline, inventory or input availability, and workforce availability. It is important to test all potential factors in your models to understand which factors, and at which grain, affect your business; there may be some that you don’t even know about yet!
When Should I Use Control Data?
Control data should be used when external factors can affect the outcome of a real-world scenario you are trying to predict. This is true for all predictive analysis when trying to answer a business question. All businesses experience environmental factors that affect demand, inventory availability, costs, workforce availability, and more. Even something as high-level as overall economic trends will affect your business and need to be accounted for in a predictive model.
For example, if you operate a brick-and-mortar business, understanding the population growth in your area will be paramount to predicting demand; as the population of your target audience grows, sales may also grow. Weather is another important factor for a business operating out of a physical location, as patrons may be more likely to come to your business on warm days than in a blizzard. Adding these factors to models will allow you to understand the impact each has on your business and will allow you to plan for future changes.
For an online business, overall economic growth or decline is more suitable for predicting sales than regional changes. If an input to your business is steel, understanding how government regulations have affected steel imports in the past will allow you to plan for regulation changes in the future. These are a few simple examples of the way external factors affect businesses every day. Testing out various correlations will allow you to understand how each factor interacts to create your overall business outcome.
How Can I Connect to Control Data?
There are many online resources to source control data at your disposal. Many government-related sets can be access for free or a small fee, such as economic data from the FRED database or weather data from the NOAA database. Other types of data, like consumer marketing data, can be found through paid services like Experian. Sourcing datasets in this way is useful when you already know what data you are looking for and are comfortable building connections to these data sources through resources at your own company. You will also need to know how to transform these datasets to the correct dimensions and granularity you are looking for.
Another option is through services like Ready Signal, a platform that we’ve developed to source and deliver various control datasets. Users can start from scratch or receive dataset recommendations based on a type of analysis and industry. Then, datasets can be automatically transformed based on geographic area, timeframe, time granularity, through data science treatments like leads, lags, and decays, and exported to a variety of destinations for your model in real time. Ready Signal also provides the Auto Discovery tool, which is a patent pending technology that allows you to upload the target variable you wish to explain and the tool will automatically identify correlated control features to test and train your models, saving you the time and effort of having to know which external factors may be interacting with your business.
One example of this process, that we’ve seen firsthand in the Food Service industry. When predicting sales without control features, our model developed with internal data only had a 16.7% error. When control features identified by the Ready Signal Auto Discovery process were included into the model, the error was reduced to 6.9%. That’s a 60% increase in accuracy achieved through the incorporation of control data where it counts.
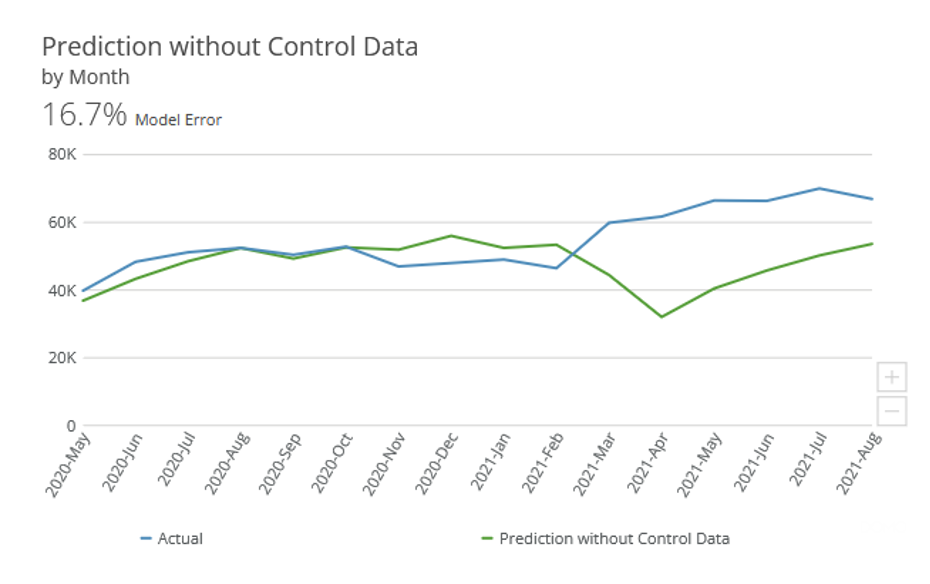
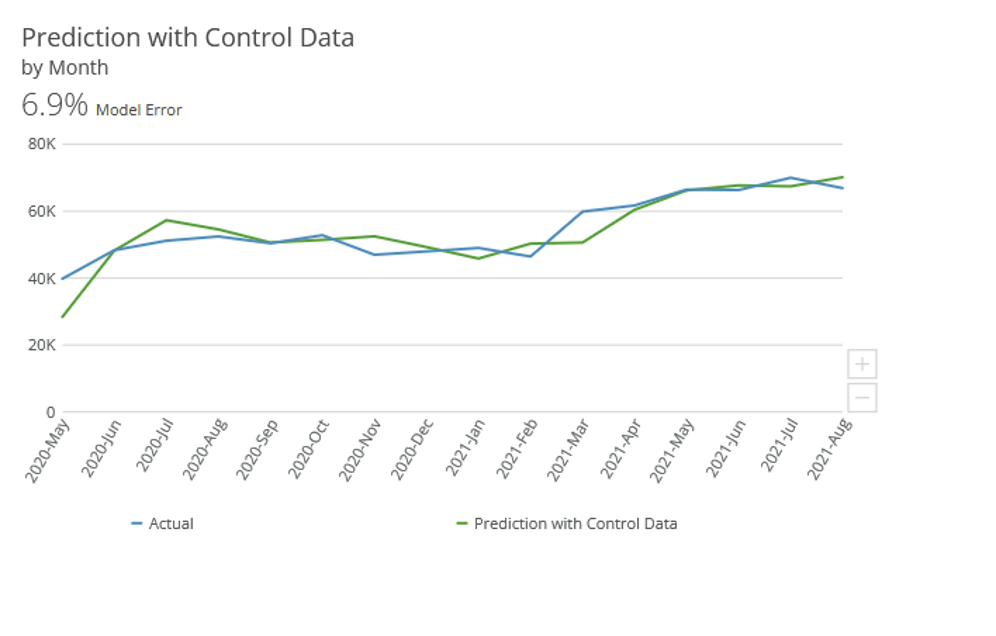
If you are looking to incorporate control data into your models and are interested in Ready Signal’s services, learn more by visiting https://readysignal.com/ or emailing [email protected].