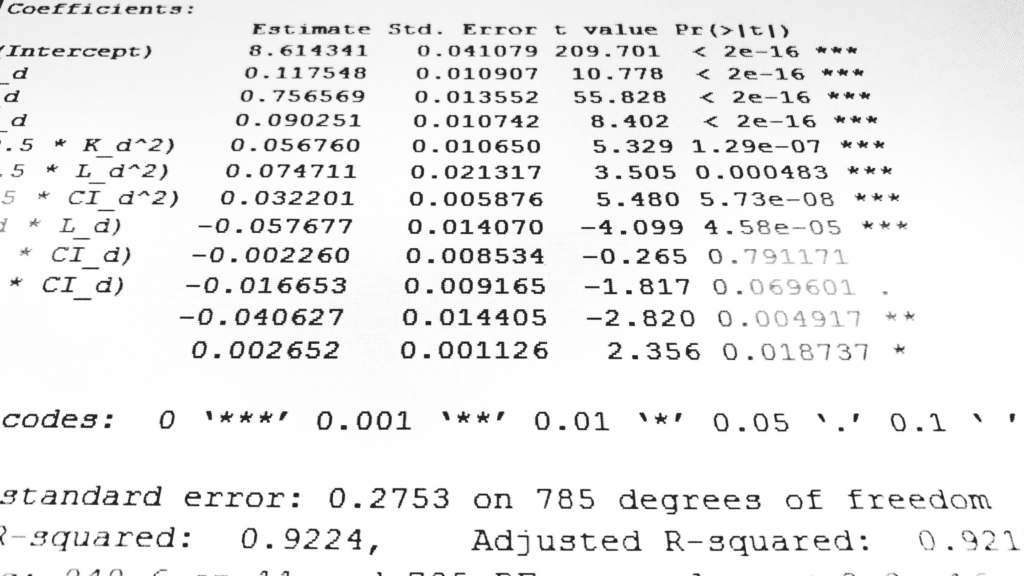
Econometrics is the use of statistical and mathematical models to develop theories or test existing hypotheses in economics and to forecast future trends from historical data. It subjects real-world data to statistical trials and then compares the results against the theory being tested.
Econometrics can help you understand the complex relationships between economic variables, such as income, consumption, inflation, unemployment, growth, productivity, and more. It can also help you evaluate the effects of policies, interventions, or shocks on the economy. Econometrics can also be used to try to forecast future economic or financial trends based on historical data and current information.
Econometrics relies on techniques such as regression models and null hypothesis testing. Regression models are used to estimate the relationship between a dependent variable (the outcome of interest) and one or more independent variables (the factors that influence the outcome). Null hypothesis testing is used to assess whether the estimated relationship is statistically significant—that is, it appears to be unlikely that it is due to chance alone.
For example, suppose you are interested in the relationship between the annual price change of the S&P 500 and the unemployment rate. You can collect both data sets for a given period and use a regression model to estimate how much the price change depends on the unemployment rate. You can then use a null hypothesis test to see if the estimated coefficient is significantly different from zero. If it is, you can conclude that there is a statistically significant relationship between the two variables.
However, econometrics also has its limitations and challenges. As with other statistical tools, econometricians should be careful not to infer a causal relationship from statistical correlation. Correlation does not imply causation, and there may be other factors that affect both variables or that cause reverse causality. For example, it may be that higher unemployment causes lower stock prices, but it may also be that lower stock prices cause higher unemployment, or that both are influenced by a third variable, such as consumer confidence.
Another challenge in econometrics is to choose the appropriate model specification and estimation method for the data and the question at hand. There are many types of regression models, such as linear, nonlinear, logit, probit, panel, time series, etc., and each has its own assumptions and properties. Choosing the wrong model or method can lead to biased or inconsistent estimates, or to misspecification errors.
What are some examples of econometric models?
Some of the common econometric models are:
– Linear regression models: These models assume that the dependent variable is a linear function of one or more independent variables plus an error term. For example: Y =α+bx+e
– Generalized linear models: These models extend the linear regression models by allowing for several types of dependent variables (such as binary or count) and different types of error distributions (such as Poisson or binomial). For example: Log(Y)=α+bx+e
– Probit and logit models: These models are special cases of generalized linear models that are used for binary dependent variables (such as yes/no or success/failure). They use different link functions (such as probit or logit) to relate the probability of the dependent variable being one to the independent variables. For example: P(Y=1) = F(a + bX)
– Tobit models: These models are used for censored dependent variables (such as zero or positive values only). They combine a probit or logit model for the probability of observing a positive value with a linear regression model for the positive values. For example: Y* = a + bX + e; Y = max(0, Y*)
– ARIMA models: These models are used for time series data that exhibit autocorrelation (such as GDP (Gross Domestic Product) or inflation). They use autoregressive (AR), moving average (MA), and differencing (I) terms to capture the dynamics of the data. For example: Yt = c + pYt-1 + qet-1 + et
– Vector autoregression models: These models are used for multivariate time series data that exhibit interdependence (such as exchange rates or interest rates). They use a system of equations to capture the relationships between the variables. For example: Yt = c + AYt-1 + et
– Cointegration models: These models are used for non-stationary time series data that have a long-run equilibrium relationship (such as consumption and income). They use a combination of differencing and regression to test for the existence and estimate the parameters of the cointegrating vector. For example: Yt – Xt = a + bt + et
Moving from the discussion of various econometric models, let us delve into the distinctions between linear and generalized linear models.
What is the difference between linear and generalized linear models?
Linear models are a special case of generalized linear models (GLM), which allow for more flexibility in modeling different types of response variables and error distributions.
Linear models assume that the response variable is a linear function of one or more independent variables plus an error term that follows a normal distribution. For example: Y = a + bX + e
Generalized linear models extend the linear models by allowing for several types of response variables (such as binary or count) and different types of error distributions (such as Poisson or binomial) from the exponential family. They also use different link functions (such as probit or logit) to relate the expected value of the response variable to the linear predictor. For example: log(Y) = a + bX + e
The main advantage of generalized linear models over linear models is that they can handle situations where the response variable is not continuous or unbounded, or where the error term does not follow a normal distribution. For example, generalized linear models can be used for modeling binary outcomes (such as success or failure), count data (such as number of events), or proportions (such as rates or probabilities).
Now that we have covered different econometric models and the distinctions between linear and generalized linear models, let us shift our focus to understanding the disparities between probit and logit models.
What is the difference between probit and logit models?
Probit and logit models are two types of generalized linear models that are used for binary response variables (such as yes/no or success/failure). They differ in the choice of link function that relates the probability of success to the linear predictor.
Probit models use a probit link function that assumes that the error term follows a standard normal distribution. The probit link function is defined as: P(Y=1) = F(a + bX), where F is the cumulative distribution function of the standard normal distribution.
Logit models use a logit link function that assumes that the error term follows a logistic distribution. The logit link function is defined as: P(Y=1) = [1 + e^-(a + bX)]^-1, where e is the base of the natural logarithm.
The main difference between the probit and logit models is that the probit model has a more symmetric shape and thinner tails than the logit model. This means that the probit model is more sensitive to extreme values of the predictor variables than the logit model. However, in most cases, the results of probit and logit models are remarkably similar and the choice between them depends on the preference of the researcher or the availability of software.
Beyond model selection, econometricians must possess a robust understanding of economic theory and statistical methods to navigate the complexities of their analyses. They also need to be aware of the potential sources of error and uncertainty in their analysis, such as measurement error, omitted variables, multicollinearity, heteroskedasticity, autocorrelation, endogeneity, etc., and how to deal with them using appropriate techniques.
Econometrics is a powerful tool for economic analysis and decision making. It can help you uncover hidden patterns and relationships in data, test economic theories and hypotheses, evaluate policies and programs, and forecast future scenarios. However, it also requires careful application and interpretation, as well as critical thinking and judgment.
If you found this article on econometrics intriguing, you’ll likely be equally captivated by our companion piece on another facet of this dynamic field. Dive deeper into the world of econometrics and discover even more valuable insights by checking out our related article.